My research synergistically combines theoretical and empirical work to get a deeper understanding of the interactions between ecology and evolution in (mostly) insect and plant systems over different time scales. The common currency of ecology and evolution are birth and death processes. They determine the population growth rate (ecological entity) which is considered an indicator of fitness (evolutionary entity) because it determines gene transmission to future generations. Specifically, I quantify ecological drivers of population dynamics, which are key components of evolutionary landscapes. This knowledge is crucial for predicting immediate population consequences of environmental change such as global climate change and introductions of novel species as well as for designing effective population management strategies. Over longer evolutionary time scales I am interested in assessing what phenotypic traits (life history and behavioral traits) should be associated with a particular evolutionary landscape. One successful approach to this problem is the development of optimization models that assume that natural selection selects for genotypes that maximize their fitness.
My work spans three broad areas of inquiry.
-
Insect and plant demography:
I use stage structured population models to identify key factors shaping population dynamics. Since environmental drivers can vary in space and time I also explore how spatio-temporal dynamics influence life-history evolution and species persistence. -
Optimal decisions in behavior and life history:
I use mathematical models predicting ultimate causes for the evolution of specific phenotypes. -
Population management:
I use a range of mathematical approaches to evaluate management strategies.
1. Insect and plant demography
A. Biotic Drivers of Population Dynamics
The Eurasian thistle, Cirsium vulgare (Savi) Ten, is an invasive species worldwide, but in western tallgrass prairie in eastern Nebraska it has remained at low densities for over 200 years. The leading hypothesis is that pre-adapted, specialized floral insect herbivores from a similar, synchronously flowering, native congener (C. altissimum, tall thistle) have included theintroduced thistle in their food range; documented reductions in C. vulgare seed production in the presence of native herbivores exceed 90%. Despite the large effect on seed production, floral insect herbivory alone is not sufficient to explain C. vulgare scarcity (Tenhumberg et al 2008). Native insects not only affect thistle fecundity but also plant survival, particularly seedling survival (Eckberg, Tenhumberg, and Louda 2012). A matrix population model predicted that this observed herbivory would result in a reduction of the asymptotic population growth rate from an 88% annual increase to a 54% annual decline (Eckberg, Tenhumberg, and Louda 2014). Interestingly, there was large spatial heterogeneity in the effect of foliage herbivory suggesting that in some locations C. vulgare could be thriving. Further, if insect herbivory is the dominant factor restricting the growth and spread of the introduced thistle then we would expect the closely related native thistle to occur in similarly low densities. On the contrary, the native thistle is highly abundant throughout Nebraska (Tenhumberg, unpublished data) even though the effect of floral herbivory on the native thistle is similarly severe. One obvious factor that might explain this discrepancy is interspecific competition. The native prairies consist of dense vegetation, and it is likely that competition for light, water, and nutrients would impact the performance of the introduced thistle species. In contrast, it is reasonable to assume that the native thistle is less affected by competition as a result of a long evolutionary history with the native prairie plants. Similarly, it is possible that native thistles have evolved some degree of tolerance to floral and foliage herbivory of native insects that is missing in introduced species. To test this hypothesis, we used integral projection models to synthesize experimental data on plant vital rates for a range of herbivory and competition levels to predict the combined effect of competition and insect herbivory on the population growth rate of the native and introduced thistle species. As expected the model predicted that the introduced thistle was more affected by competition with the native plant community and by native insect herbivory than the native thistle (Tenhumberg et al 2015).
Tomomi Suva hugging C. altissimum
B: Spatio-temporal dynamics
Demographic rates (survival, growth and fecundity) vary in space and time, and this variation can be important for life-history evolution and species persistence. We are exploring spatio-temporal dynamics by analyzing long-term demographic data sets and by constructing mathematical models.
Spatial dynamics:
Many demographic studies combine data from different locations to increase sample size. This approach assumes that populations are well connected via dispersal. However, if there is dispersal limitation this spatial averaging can mask relevant local dynamics and lead to erroneous conclusions about the fate of population viability. Such dispersal limitation may be the result of habitat fragmentation isolating subpopulations even at relatively small scale. This is especially important if biotic and/or abiotic factors vary between habitat fragments. For instance, we have demonstrated that foliage and seed herbivory of C. vulgare can vary dramatically at relatively small spatial scale in Midwestern grassland prairies (Eckberg, Tenhumberg, and Louda 2012). Native prairies are particularly severely impacted by habitat fragmentation. For instance, in North America over 99% of the tall grass prairie has been cleared resulting in extreme habitat fragmentation. One example where the aforementioned averaging results in misleading estimates of population viability is Opuntia macrorhiza. We reanalyzed a seven-year long data set (Keeler and Tenhumberg 2011) from five plots in Boulder County, Colorado, USA. In the study area the distribution of this species is sparse (only 6% of the vegetation consisted of O. macrorhiza) with infrequent dispersal between plots. The predicted stochastic population growth rates and the spatial arrangement of the plots suggested source-sink dynamics with three subpopulations shrinking between 2-39% annually depending on the distance to the source populations. The loss of the largest source population would likely result in the extinction of O. macrorhiza in this area, which contradicts the prediction of the averaged model (population stasis, Keeler and Tenhumberg 2011). I am also involved in collaborations using mathematical models to understand the dynamics of patches that are linked via dispersal (metapopulation models, Roberts et al. in progress, Callahan et al. 2015, Eager et al 2013, Tenhumberg et al 2004).
Opuntia macrorhiza (photo credit: Kathy Keeler)
Temporal dynamics
In insects, temperature is particularly important because it influences not only survival, fecundity and growth, but in many cases temperature also determines the number of generations per year. Even within the normal range temperature can impact mortality indirectly via its effect on developmental time because daily survival is multiplicative. We explored this indirect effect on the population dynamics of the western corn rootworm (Diabrotica virgifera virgifera LeConte, Haridas et al 2016), an economically important pest species in corn. The advantage of using pest species as case studies is that there are ample data available to estimate life history parameters. In our case we even found published field data to estimate density dependent juvenile survival (overwintering egg to the adult stage) providing an assessment of variation in survival in the field unrelated to temperature (e.g. variation in predation pressure, storm related drowning of early juvenile stages). Our model suggested that boom and bust population dynamics can be explained exclusively by small variation in maximum daily survival (93.5-95.1%) and the indirect effect of variation in temperature.
Diabrotica virgifera virgifera LeConte
(photo credit: Lance Meinke)
We are interested in answering questions related to temporal variation in abiotic drivers of population dynamics. A. scaphoides is an iteroparous, perennial legume that is endemic to high-elevation sagebrush steppe in a small area of southwest Montana and adjacent parts of Idaho. This plant species has been monitored continuously since 1986, which makes it one of the longest data sets for perennial wildflowers in the USA. I joined the long-term monitoring project in 2014 and in 2015 Elizabeth Crone (Tufts University) handed it over to Drew Tyre (Nebraska, SNR) and I.
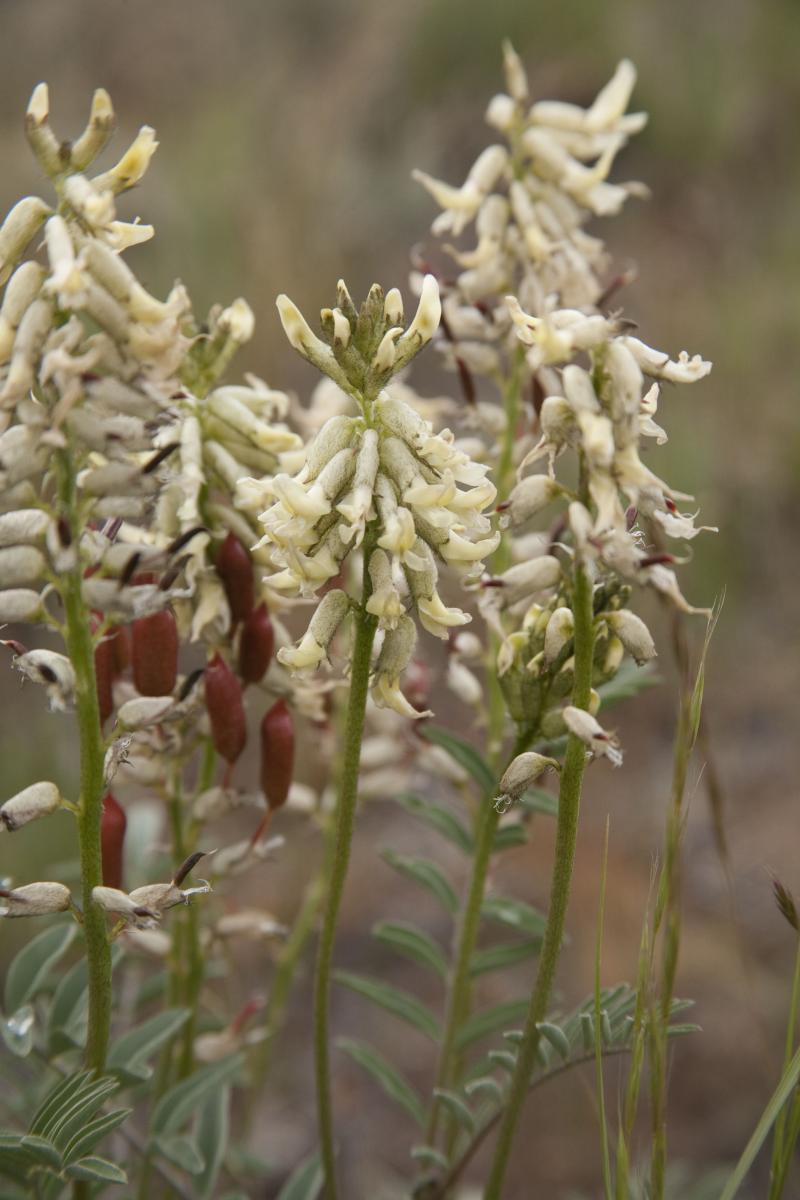
A. scaphoides (photo credit: Drew Tyre)
A. scaphoides monitoring site
(photo credit: Drew Tyre)
2. Optimal decisions in behavior and life history
I use optimization models, in particular stochastic dynamic programming (SDP) models, to understand the evolution of life history strategies and behavior. This modeling approach assumes that natural selection selects for behavior and life history strategies that maximize fitness. In many cases optimal strategies are not fixed but rather depend on internal and external states. For instance, internal states could be age, energy level of predators, and egg load in parasitoids; external states could be density and distribution of resources, or predation risk. Thus, instead of predicting static optimal strategies, we are interested in understanding the evolution of norms of reaction which could partially explain phenotypic variation in strategies. The overall fitness payoff depends on the cost and benefit associated with a particular strategy (e.g. the trade-off between current and future reproduction). We have used stochastic dynamic programming models to address a range of questions, including optimal age and weight at maturity (Tenhumberg et al. 2000), optimal patch leaving behavior (Tenhumberg et al. 2001), optimal time allocation between searching for hosts and food (Tenhumberg et al. 2006), and optimal resource allocation to growth and reproduction (Miller et al. 2008). Furthermore, we have used game theoretical approaches to predict optimal life history decisions if the payoffs are frequency dependent (Bouskila et al. 1998).
Colton Watts used this modeling approach to evaluate how the trade-off between foraging for food and avoiding predation shapes the daily foraging pattern of web building spiders. His model predictions qualitatively matched observed foraging patterns of spiders in the field (low energy reserves) and in the lab (high energy reserves) (Watts et al., in manuscript). Shivai Jadeja constructed a model predicting the optimal flower retention of sequentially flowering plants; the model predictions were consistent with the results of her field experiments on yucca plants (Jadeja and Tenhumberg, in progress).
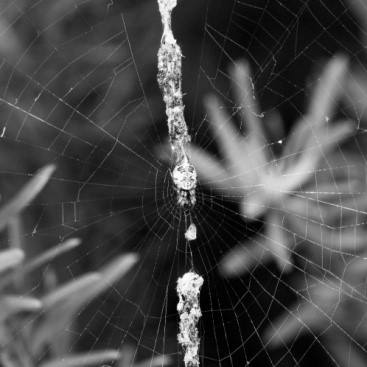
Cyclosa turbinata (photo credit: Colton Watts)

Yucca glauca (photo credit: Drew Tyre)
3. Population Management
There is a long tradition of using mathematical models to evaluate the efficiency of different management strategies. For instance, models can identify the vital rate (e.g. changing survival or fecundity) managers should target to get “the most bang for the buck”, which is particularly important when resources are limited. The prevailing method is sensitivity or elasticity analysis which explores how much the predicted population growth rate changes with very small changes in parameter values (e.g. Tenhumberg et al 2008). We have used numerical (Monte Carlo methods) and analytical methods (robustness analysis) to predict the effect of large changes in multiple life history parameters to analyze different systems ranging from invasive to endangered species management (Deines et al. 2007, Lubben et al., 2008, Tenhumberg et al 2008, Lubben et al, 2009, Miller and Tenhumberg, 2010). The lion’s share of models aiding population management focus on ecological entities (population growth rate, population size) but for some applications the speed of evolutionary change is also of concern. Due to the lack of new herbicide sites-of-action and increased reports of herbicide-resistant weeds, resistance management has become one of the most concerning topics in agriculture. Some of my recent projects assessed the potential of management strategies to slow the evolution of herbicide resistance in economically important weeds (Werle et al., in review) and of pesticide resistance in pest insects (Bacillus turingiensis proteins expressed in transgenic plants, Haridas et al, in review).
I am collaborating with Mathematicians (mainly Richard Rebarber, Department of Mathematics, Nebraska and Stuart Townley, University of Exeter, UK) to persue a new exciting research direction is exploring the utility of optimal control theory, common place in engineering, to addressing questions in population management. The idea is that population mangers can manipulate some terms in the biological system in order to obtain a desired response such as minimizing the cost associated with the presence of a desired or undesired organism. In case of pest species, the cost may consider the loss in harvest associated with a specific infestation level of the pest and the cost of insecticide applications or biological control agents (Edholm et al, PhD thesis 2016). The magnitude of control can vary over the season for instance to account for temporal effects of the pest on harvest. The next step is to consider manipulating the spatial spread of pest species by using spatial variation in control strategies. ecological systems are unlike engineering systems with highly stochastic dynamics and parameter estimates influenced by both process and observation error. This might limit the utility of these methods to population management. In order to control a system robustly, it might be better to use feedback control, that is, the control is a function of the state of the system at each time step. For example, managers can remove or add members of the population (Integral control: Guiver et al. 2015), or change stage specific vital rates such as insect larval survival (Adaptive control: Guiver et al. 2016). The next step is comparing the performance of optimal control to feedback control under different scenarios of stochasticity (increasing variance in observation error and/or process error).